Social, Cognitive, and Behavioral Science
When will a social media protest transition to a civil crisis? What is the tipping point for rhetoric to become action? How do we design effective, massive health messaging campaigns to make the public’s health more robust? Effective analysis and understanding of such questions must do more than compile data; it should be driven by theoretical knowledge and first principles of what motivates human behavior. This implies multiple levels of scale that coincide with key disciplines of human behavior—the cognitive, behavioral and social sciences—each of which offer unique insights into understanding and prediction. Our sophisticated computational simulations are designed to account for such theoretical first principles, allowing us to provide decision-makers with robust predictions of the influence of policies and the specifics of their implementation on human conduct.
The simulation of systems that implicate humans in any way requires some consideration of the core foundational disciplines that study human behavior (e.g., cognitive science, psychology, sociology, communications, economics). Naturally, because we deal in simulations, a central question arises: What kind of formal abstractions are both feasible and useful for the problem at hand, and implementable in a simulation environment? Social abstractions might use graph dynamical systems theory; abstractions in respect to individual- or team-level behavior might leverage cognitive architectures or precise psychological measurements. Our team employs a set of methodological perspectives that are designed to ground our simulation approaches in cognitive, behavioral, and social theory. For example, we conduct human experiments in controlled social settings to understand decision-making and resulting actions. We develop sophisticated measures of attitude formation (the automaticity perspective from Rich Fazio’s groundbreaking work) that can be deployed on Amazon Mechanical Turk. We’ve developed a technical simulation platform, The Matrix, that is designed to integrate cognitive models of human behavior (from cognitive science and cognitive psychology) with theory from the social sciences (e.g., social networks, behavioral economics) to drive the simulation of at-scale human systems.
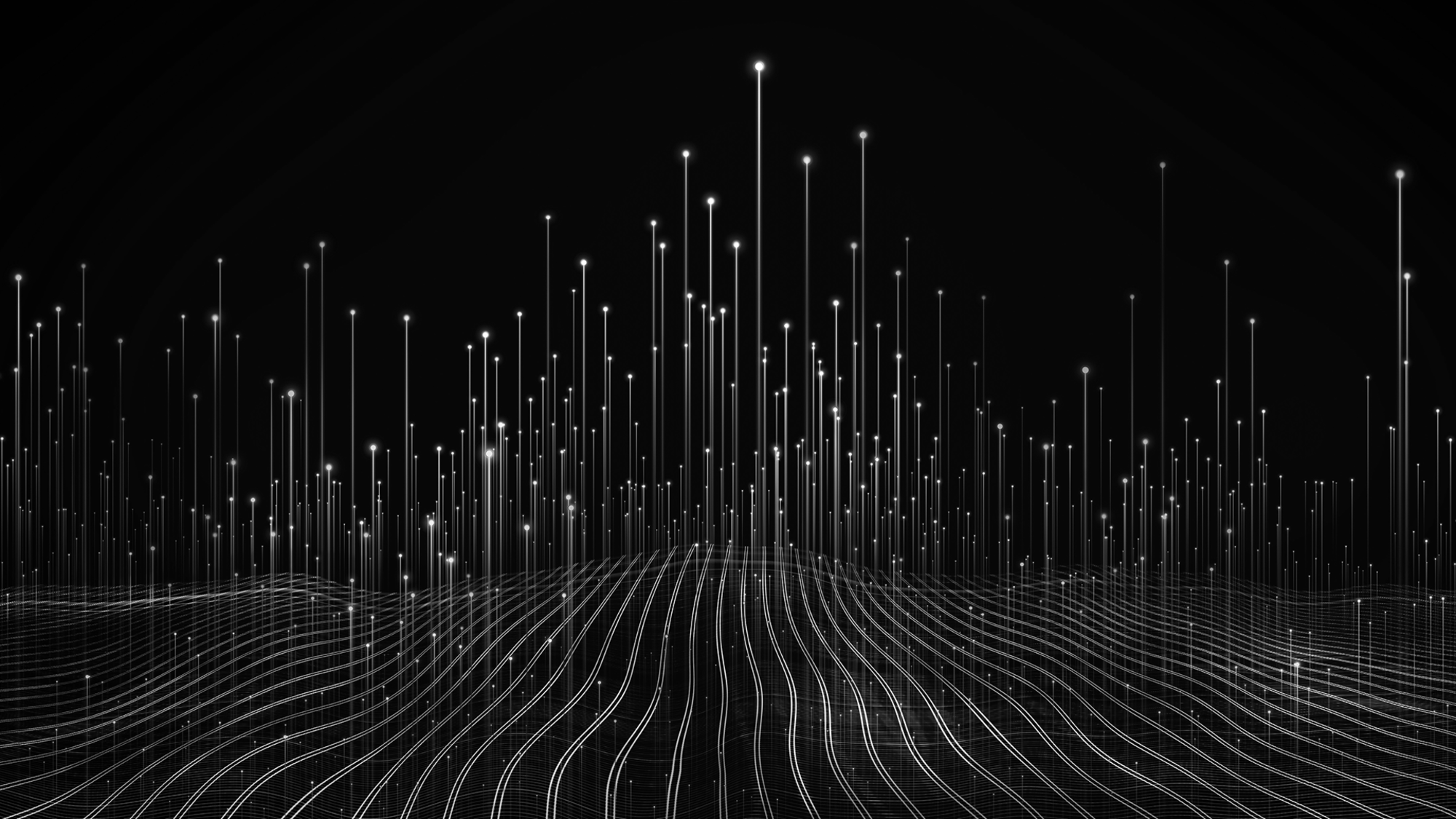